Nowadays, it seems everyone has an online store. If you’re an e-commerce professional, you’re probably always on the hunt for the latest best practices and cutting-edge technology that will boost your conversion and enhance your customer experience, helping your sales soar.
But what exactly do you know about the inner workings of e-commerce site search? How does it “think” and “behave”? How is it different from a regular content-based search, such as the one conducted on Google, Bing, or Yahoo?
According to Forrester, 43% of online shoppers go directly to the search bar when landing on an e-commerce website. And when those searches are fruitful, conversion rates are three to four times higher (on Amazon, they shoot up to 6x!). Those are huge numbers that make a huge impact on your sales. Ready for another huge number? 61% of e-commerce websites perform below average when it comes to internal site search.
Understanding how e-commerce search works can directly help you maximize your online revenue. Keep reading to learn more about your online store’s internal search and what makes it so different from content searches through Google.
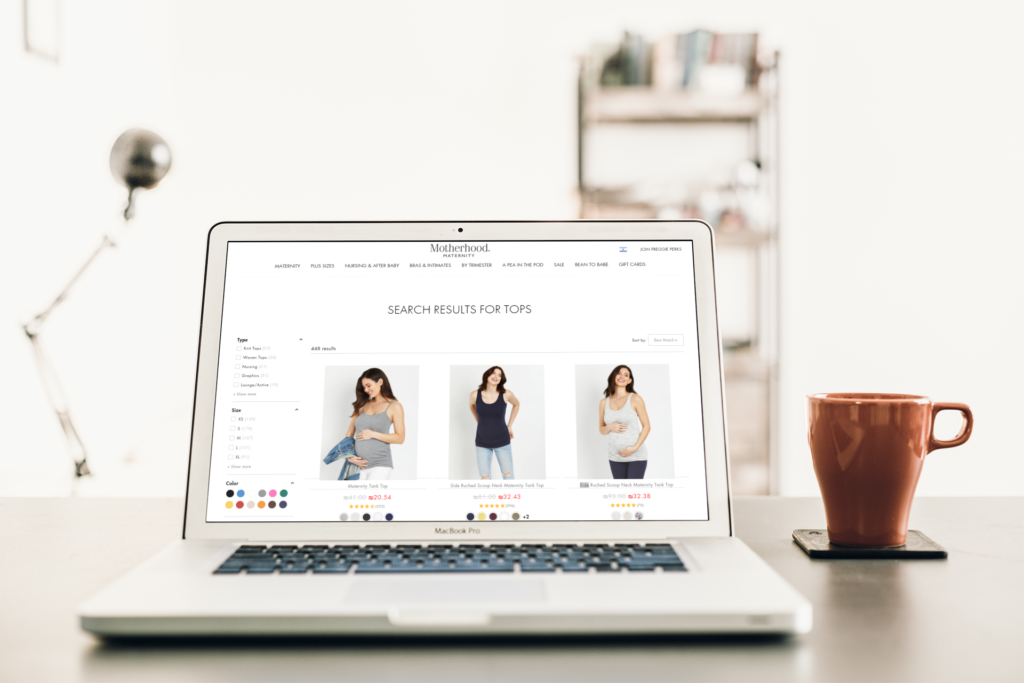
The Problems
With new technology being introduced all the time, it can be hard to keep up and prioritize the best solutions your online store needs now. However, it’s important to pause and make a game plan, because when it comes down to it, every online merchandiser or e-comm manager has dealt with some version of the same problems, over and over.
We’re willing to bet you’ve danced around all of the following issues multiple times, finding temporary fixes that didn’t quite hit the mark.
Any of these sound familiar?
- Your search results are not relevant.
This may stem from the items not being properly tagged or not being indexed. A unique problem with eCommerce is that e-comm data has to be weighed, which means that the different parameters have to be ranked in order of importance in an effort to determine the overall relevance. Therefore, the weight of the field may not be high enough for it to show up in a particular search. This issue probably leads the user to give up on your search function altogether and go scour your category pages hoping they make more sense – or, they give up entirely and bounce from your site.
- Your new arrivals can’t be found.
They’ve just landed, they’re super trendy, and everyone wants them… but no one can find them. Somehow, when the user types in the keyword that should magically make them appear in all of their glory, they’re nowhere to be found. The result? Disappointed could-have-been customers eager to jump on a trend, inhibited customer acquisition, inventory surplus, and of course, fewer sales for top-line items less likely to be discounted.
- Out of stock items are appearing
It may be the SKUs themselves, or certain colors or sizes, and they may appear in broad search results or even after using filters. Regardless of the road taken, showing shoppers items they can’t order is frustrating for them, for you, and for your bottom line.
No one wants a frustrated customer. So, to address these issues for good, get a better grasp on your e-commerce site search. Once you tame the beast, things will turn around quickly. Let’s take a closer look at how things work, starting with some common misconceptions.
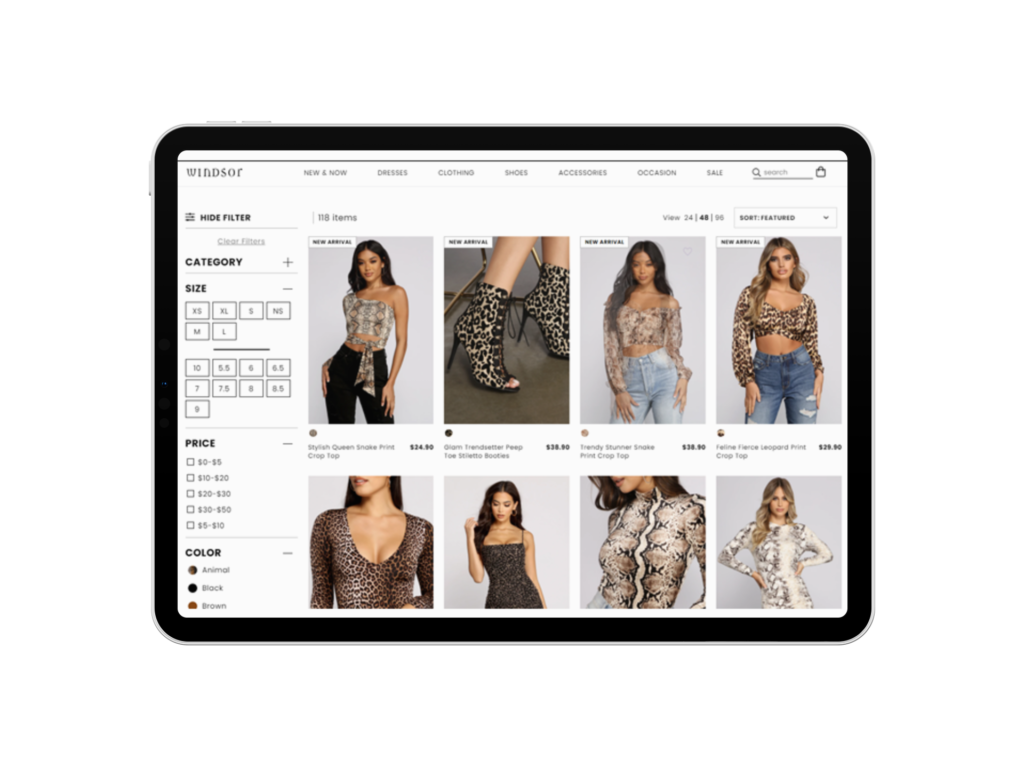
E-Comm Site Search vs Google Search
A search is a search is a search? Wrong. Content searches, like Google, were built to find relevant content, with the ultimate objective of meeting the user’s expectations. On the other hand, e-comm searches are used to find products (user’s expectation) and generate sales (merchant’s expectation). When you dive deeper, the difference becomes even more pronounced.
The Basics of Content Search
- The User Above All Else
To proceed with a content search, such as on Google, the user first needs to start typing keywords into the search bar. Google then tries to guess the rest of the query and uses autocomplete to simplify and hasten the user’s experience, while also relying on aggregate data from content provided to other users in the same location, with the same query. Once they hit Enter, the search engine yields pages upon pages of search results (hello, 8,020,000,000 results in 1.15 seconds!).
In other words, in content searches, results are unlimited. This is why for many searches, a direct answer to the user’s query is shown as the very top, pulled from Google’s knowledge graph. What ensues is, hopefully, answering the user’s query in just a few bullet points, without them even having to visit another website. These are particularly useful for users performing a voice search or browsing on a mobile device.
The main goal of a content search is to serve the most relevant results for the user query, or better yet, for the user’s intent – to ultimately please the user. Everything is automated to serve that one, clear goal, no matter which direction the user is pointing at: whether it’s navigational, informational, or transactional, their satisfaction is measured through engagement.
The content search engine measures engagement using metrics such as the time the user spends on a particular site, their bounce rate, as well as the terms entered following that initial search, which serves to indicate whether the search was successful.
For example, if a user was looking for a recipe, the search will notice that the user only spent 5 seconds on a particular website, hinting that it was not the winning recipe, as opposed to 20 minutes on one they likely deemed satisfactory. This data is then collected for every language, country, and industry, and compared to determine quality, relevancy, and satisfaction in order to refine future searches more successfully.
- Relevancy
Google Search’s mission? “To organize the world’s information and make it universally accessible and useful.” Not an easy task! To do so, crawlers analyze web pages and visit their links, much like a user would. They then store information related to those pages and take notes of signals, including keywords, external links and page freshness, to index them. And because websites are always being updated, pages will be crawled again, noticing changes and indexing new information.
The objective here is relevancy. And since Google searches through many data streams with hundreds of signals, all automatically optimized for the user, localization and personalization (think search history) also play a huge role when it comes to relevancy.
Here’s what that can look like, in practical terms. Looking for a restaurant? The search will show dining spots near the user, unless they specify another location in their query. For every search, there could potentially be millions of matches. That’s why content search makes decisions based on what it knows about you, as well as previous data collected on a particular subject, in order to show you things it believes to be relevant to you.
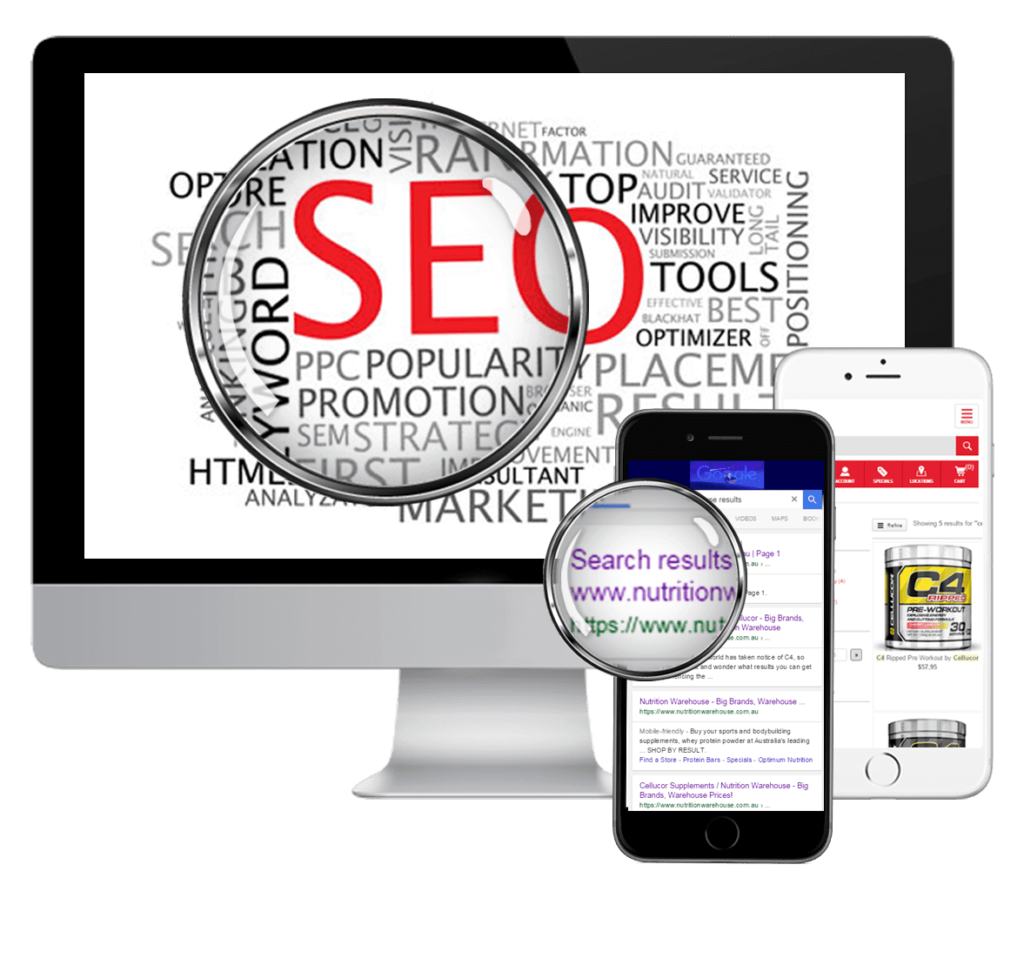
- Ranking the Content
But how is content ranked on Google? Google doesn’t need to adapt its search to every store, but it does have to deal with the topic of competitiveness since it’s the main space where businesses battle for users’ attention online, which also means it’s subject to algorithm manipulation. Information is ranked based on a wide variety of factors, such as search keywords, page contents, source expertise, location, external links, and language. Depending on the user’s search, the weight will be applied differently to each factor. The freshness identified by crawlers can also be relevant here, although is more likely to really matter for news-related searches.
For relevant results to be found, content search first has to identify the user intent. To do so, it will analyze the keywords entered, correcting them or using semantically close queries if need be, to come up with relevant pages. It will then analyze the content it is coming up with to make sure it relates to your query. Keywords are important, of course, but are not the be-all and end-all. That’s why the page’s entire content is analyzed, making sure it is relevant to the user.
Now that relevant content has been identified, it has to be effectively ranked. This ranking will depend on how trustworthy, authoritative, and knowledgeable a source is known to be. This analysis often heavily relies on links. Do prominent sites refer or link to the content? If so, it’s bound to rank better in search results, but Google still ensures that the right balance of authoritativeness and balance is achieved so that results are not swayed. And if a few websites are neck and neck? User experience will prevail, with content deemed more accessible ranked higher, whether that means it’s available in a mobile version or loads faster.
- A Constant Analysis
With content search, there will always be results shown, whether or not they are exactly what the user was looking for. If the end-user looks through the results provided but doesn’t deem them satisfactory, they won’t know whether it’s because the content they were looking for doesn’t exist, they didn’t use the right keywords, or the topic is not covered by Google. And since the user has very limited filter options to play with, they will have to start a new search to zone in on what they were originally looking for.
But since Google is so smart, it’ll notice the subsequent search and take note of it, analyzing it to optimize relevancy on a particular topic. Moreover, because it relates search terms to one another, it’s able to come up with a list of related questions (under “People also ask”) that may help the end-user refine their search and find what they were looking for without having to start their search all over again.
But Google doesn’t know it all—it learns as it goes. In fact, 15% of daily searches are ones Google hasn’t encountered yet! That’s why it needs to rely on a multitude of factors to make sure the user is satisfied.
Google Search also goes through extensive testing and quality checks to ensure that, even though its results are automated, they’re up to humans’ expectations. And to match those expectations, the content is shown in the most useful way possible, with different types of results usually merged in a single result stream, like images or videos presented within a textual search, along with Google-owned integrated apps or partner features when applicable. Wondering how to install a drain pipe? You may see a YouTube video. Looking for the weather in a particular town? You’ll see the forecast. Want to know how to get somewhere? You’ll see a map. Intuitive, useful, balanced, relevant.
E-Commerce Searches
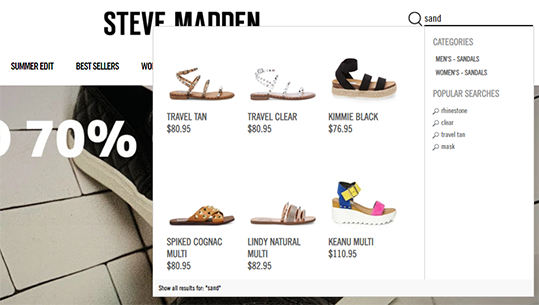
Unlike content searches, which are optimized per individual user, e-commerce search has to meet the needs and expectations of two personas: the shopper and the merchant.
With e-commerce searches, there’s always an outcome in mind. While the user’s (customer’s) happiness is still of utmost importance, a wide array of other factors also come into play to ensure that a sale takes place and that the customer comes back. Whether it’s AOV (average order value), CR (conversion rate), profit margin, RPV (revenue per visitor), inventory management, or customer satisfaction, merchants certainly want shoppers to buy (of course!); but more specifically, merchants want customers to buy what they most want to sell.
Optimizing eCommerce Search Results
Since eCommerce search needs to hit various object functions for optimization, it must provide a number of vehicles to achieve these goals. These vehicles can include fully automated AI or algorithms to optimize, rule based, or manual means. Merchants would use one or more depending on their needs, traffic, use cases, and skill-set.
eCommerce Results Set
eCommerce results sets form part of a finite catalog – unlike the endless results set harvested by Google. This means that the underlying search technology is different, and also, eCommerce merchants and shoppers can much better scrutinize the relevancy of ecommerce search results. Merchants know their inventory, styles, and products well, sometimes by heart. They know that a product should be found for certain queries, and if it isn’t – it necessarily points to a problem.
The same goes for shoppers. If they were enticed by a marketing campaign, followed the link, and then couldn’t find a product – they will be understandably frustrated. On the other hand, after conducting a Google search, most users would not know whether their desired result was not found due to an issue with relevancy or indexing, or if the content they’re looking for simply does not exist.
Searchandising
One way to optimize search results is through online merchandising for search, sometimes called searchandising, which directly impacts the user’s experience and the retailer’s results. Just like the creation of category pages, search result pages can also be merchandised to drive more sales to the items that benefit the store most.
Without searchandising, search results may innocently end up consistently irrelevant. For example, take a site selling state-of-the-art drones. When searching, a user may encounter a drone propeller as the first result, due to its popularity and relevancy. However, a merchandiser may want to inspire the visitor by first showing a full-fledged drone, and only then displaying results for parts.
Effective searchandising can also prevent last season’s styles or the most unpopular (read, least clicked) designs and colors from showing up first, turning off a potential customer from wanting to continue browsing. On their own online boutique, a retailer can choose to prioritize items whose sale benefits them most, like new arrivals or high-profit items, at the top of search results.

Personalization
Despite what you may think, content search engines don’t utilize personalization signals much, beyond location and immediate context. This limited use of personalization differs from the impressive capabilities in ECommerce site search, which based search results on – among other parameters – recent searches and recently browsed products. This use of personalization can do wonders for conversion, and enhance the user experience.
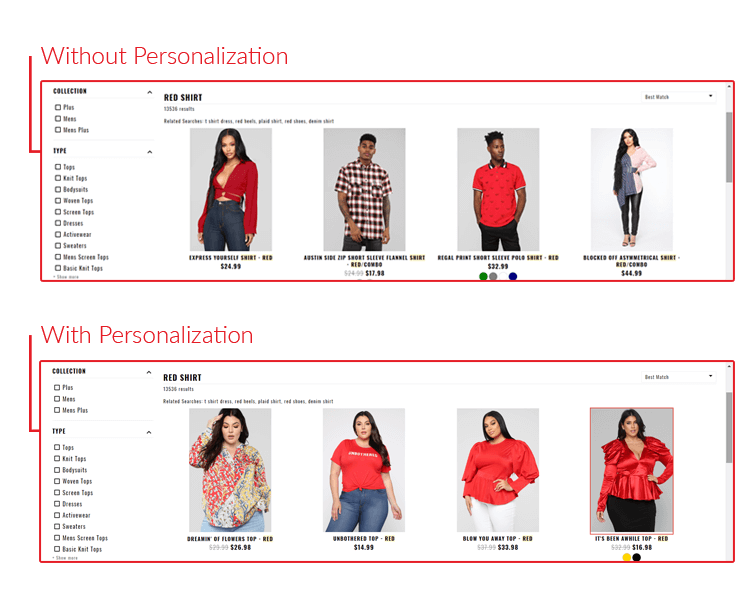
Predictive Search
While content search offers auto-complete for queries, ecommerce sites suggest both queries and products based on the first few strokes of a user’s keyboard in the search box. This proactive approach can save a user time typing (that’s especially important for users on mobile devices where typing is slower and more difficult), and potentially also steer him or her to the products they had in mind more effectively. Since the auto-complete function is visual, the seller can proceed directly to a product page from the search box, without having to pass through a results page.
Without this technology, users would have no indication of their search’s success chances before hitting ‘Search’, and if their search came up empty they could either abandon altogether, or embark on a trial and error session with the search box. Both options do not bode well for user satisfaction.
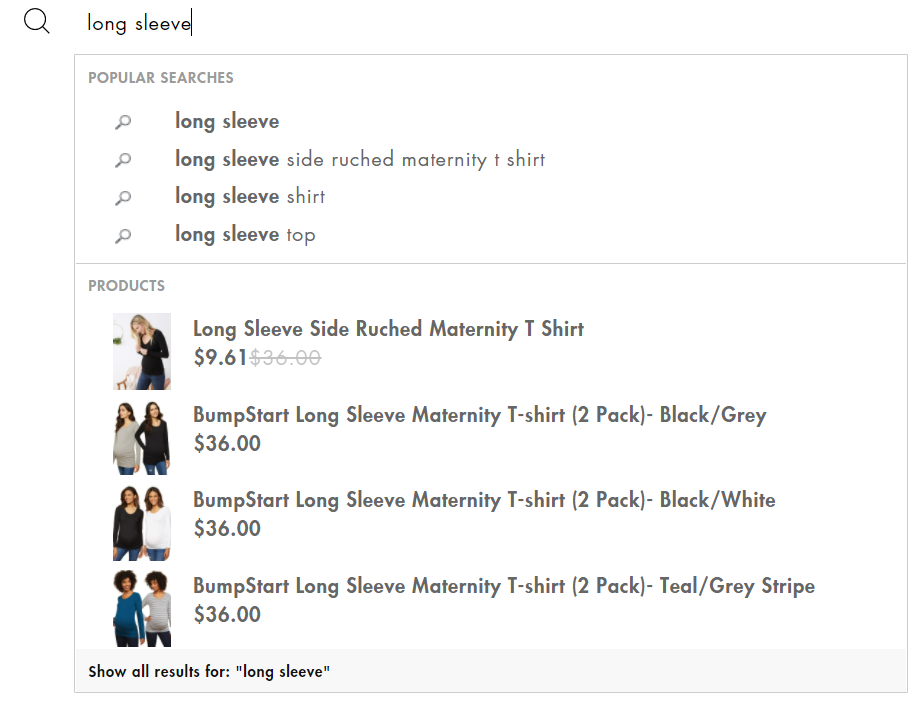
Visual Discovery
This is another form of merchandising that can be highly effective at inspiring shoppers and driving traffic to a merchandiser’s chosen items: a user clicks into the search box but doesn’t type a thing, to be met with a drop down depicting various items’ visually. This is called “visual discovery”, as it is more similar to browsing than searching; it is also sometimes called a zero-shot search, given that the user has not entered any query.

The Data
As we reviewed, content search measures satisfaction through user engagement, whereas e-commerce merchants see a sale as the ultimate sign of a successful search. The user’s intent is also usually pretty clear since the search box on e-commerce sites is mostly transactional (no surprises there!).
The user usually visits an e-commerce site with a specific idea in mind, whether it be buying a particular type of product or redeeming a special offer. And because merchants know which keywords were used during the search and are able to track the user’s journey on their website thereafter, they can quickly become aware of problems if something wasn’t found or if a popular search isn’t converting as expected, then rectify the situation either by diversifying their products, reorganizing their data, or optimizing their search through AI.
Unfortunately, catalog data is often lacking on many websites, which affects e-comm search results. Product descriptions could be missing, incomplete or simply devoid of words in the user’s vocabulary (e.g. “jumpsuit” versus “romper”; “cardigan”/“cardi”); a product identifier such as the SKU could be hidden in the style filter, in the product type, or even in the collection name, a huge issue for ecomm stores selling parts or other replaceable items; the item’s color could be directly in the product name, hidden somewhere on the PDP, mentioned using words less likely to be searched (e.g. “periwinkle” instead of “blue”), or not mentioned with words at all.
Data dimensions, variants, and attribute names vary from website to website, and rules are hard to implement. This often leads to the data being disorganized. In e-commerce searches, relevant data first has to be found, but then has to be weighed. This weighing of the information needs to be done automatically, but requires manual intervention to identify which parameters matter most to a particular retailer.
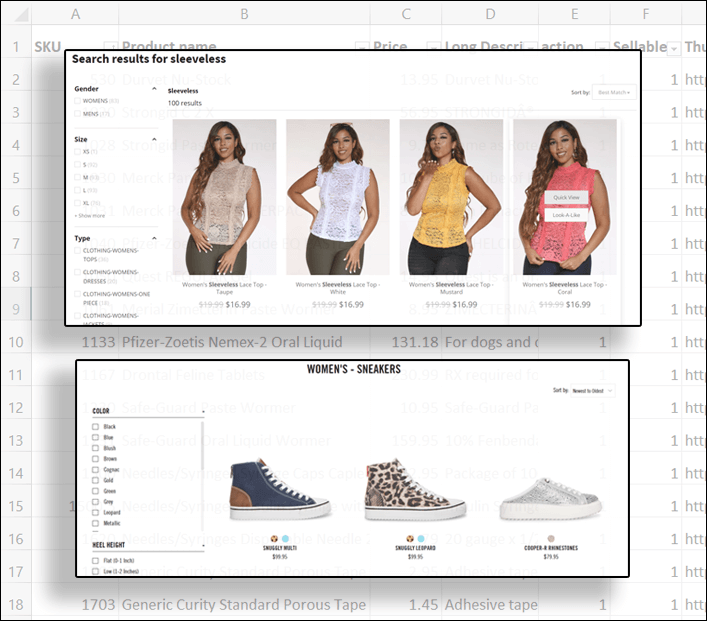
The merchandiser is responsible for tagging and indexing items. Without this data, there is no way for the e-commerce search engine to deliver relevant and effective search results. Products that have not been tagged, or that have been indexed incorrectly, could fail to show up, even when they are relevant to the search query.
Since data organization varies from one store to another, tagging and indexing can impact both site conversion and search engine traffic. Google search results and ecomm site search results may be completely different for the same query.
Given the importance of tagging, and how tedious and error-prone this manual task is, merchants can greatly benefit from automated data enrichment.
Automatic data enrichment, sometimes called hyper-tagging, leverages product catalog data to tag and index products, building on text descriptions, product images, and even external data sources such as product UTC or GTIC.
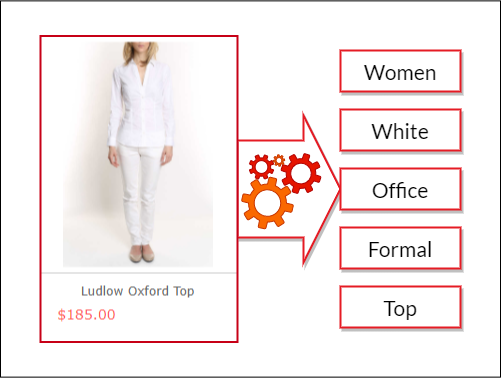
To the same end, artificial intelligence text models based on Natural Language Processing (NLP) are used to extract tags and attributes from product descriptions. Finally, the most recent advances in visual processing are being used to extract tags from product images, further reducing the merchandiser’s manual load.
The Results
Remember we said that e-commerce search is finite, unlike content search? This means that sometimes, an e-commerce search can yield zero results. This could be because of a number of reasons, including products that have been tagged or indexed incorrectly, an inferior site search tool that doesn’t recognize synonyms or typos, or the simple fact that the retailer does not carry a particular product. No matter the reason, the merchant will know that a product is missing or wasn’t found and that their data needs to be rearranged so that search results are optimized – since an empty results page is a negative user experience.
On their end, e-commerce customers can usually use a wide array of filters to find more relevant results, something they can’t really do on Google. But here’s a shocking stat: the team at the Baynard Institute has found that 84% of e-commerce sites provide a poor or mediocre filtering experience for users. And yet, filters are essential tools, helping shoppers conduct highly effective product searches. When automated and optimized, the right filters show up for the right product searches and help the customer find what they’re looking for in a timely fashion.
Both text and image information can be used to enrich the search results, so that the same catalog items can be searched through text or images. However, as opposed to content search, e-commerce search is mostly visual in the sense that the results are visual, and are usually contained within a product grid.
The Challenge
For an e-commerce search to be truly successful, two elements have to be combined: good search queries, with your customer knowing what they want and using the right keywords, and good indexed data, where the right data is available for your customer to find.
And since every e-commerce site is different, that data will look different everywhere and the site search tool used is bound to react differently. So while data may be perfectly indexed (hey, it’s plausible), the e-commerce user may not know how to conduct a successful search, or the search terms may be great, but the right products may not have been tagged using natural language – including typos and synonyms – leading to less visibility.
Google constantly works to crawl websites and rank information, creating as great a balance as possible in its search results. The great challenge of e-commerce search is that it’s every man for himself. Once on a particular retailer’s e-commerce platform, there is no other competitor than that particular store, and its strengths and shortcomings are laid bare before the user, front and center. Depending on the situation, whether it be lack of search results, a frustrating site experience, delivery fees, or something else, the shopper can easily return to Google and choose a different e-commerce store, having to, once again, try to navigate its unique quirks.
The overall design of the webpage can also affect the search. If every e-commerce website looked like Google, with the main focus on the search bar, then most people would start their visit by searching for what they’re looking for, as we already know user intent is usually clear in e-commerce search. But many retailers’ search bars are hard to find, making their search problems start before the actual search even begins.
Ultimately, these two crucial search engines function differently because they serve different goals – and different audiences. If you’ve been operating under the assumption that your site search did not matter for conversion and other KPIs, or worse, that you had no way of impacting it, we hope this guide has helped you realize the opportunity that lies in front of you. Your shoppers want to find things they love; help them.
The Bottom Line
Let’s recap what we’ve learned:
Differences between Google and E-Commerce Search
Ecommerce | |
The goal is to please the user. | The goal is to please both the user and the merchant. |
The objective is relevancy. | The objectives include, but are not limited to: relevancy, AOV (average order value), CR (conversion rate), profit margins, RPV (revenue per visitor), inventory management, and customer satisfaction. |
User satisfaction is measured through engagement. | User satisfaction is measured through sales, conversion, and engagement |
Searchable data approaches infinity. | The data is finite; part of a catalog. |
Engine auto-completes queries. | Auto-complete offers queries and products (the latter in a visual way). |
Searches through many data streams using hundreds of signals, all automatically optimized for the user, taking into consideration localization and personalization. | Searches within a limited catalog, where product data sometimes: Can’t be found due to mistakes in style filter, product type, collection name, item name, etc. Is hard to find due to wrong keywords, missing tags or indices, wrong field weight assigned and more. Data has to be weighed. |
If a result is not found, the end-user won’t know if it’s because it doesn’t exist, they didn’t use the right keywords, or the topic is not covered by Google. | If an item is not found, the merchant knows a product is missing or that their data needs to be rearranged so that searches are optimized. |
There will always be results to browse. | Sometimes, there won’t be any results to browse. |
Google doesn’t need to adapt its search to every store. | Data is stored differently from store to store, which may impact how it is found on Google, as well as on the website itself. |
Localization and personalization play a huge role in showing relevant results. | Localization and personalization only come into play when those parameters are in use on the particular online store. |
Results feature snippets (text). | Results are visual (photos), usually within a grid. |
The user has very limited filtering options. | Users have numerous filtering options available. |
User intent is navigational, informational, or transactional. | User intent is mostly transactional. Users come with a specific idea in mind. |
Different businesses compete in the same space, making content search engines subject to algorithm manipulation. | Everything is built to satisfy a specific store’s needs. |
Different types of results are merged in a single result stream (i.e. images or videos can be presented as a result of a textual search). | The same catalog items can be searched through text or images. Both text and image information can be used to enrich the search results. |
E-commerce and content search are both crucial for running an online business, but it’s important to give both of them the personal attention they deserve to truly optimize results. By understanding the differences between e-commerce and content search, data can be shaped and searches can become more fruitful – and profitable.